European Union’s Horizon Europe research and innovation program
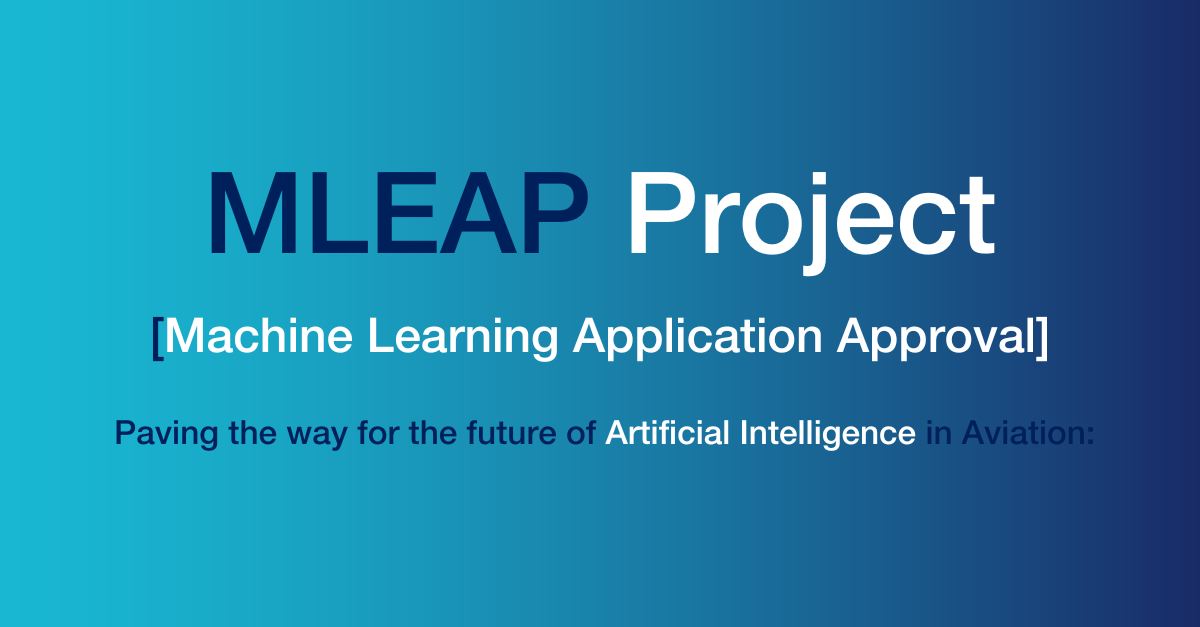
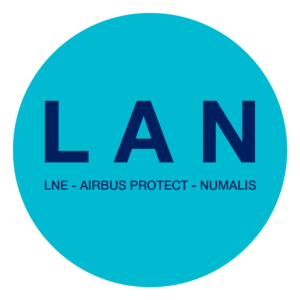
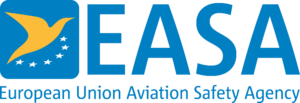
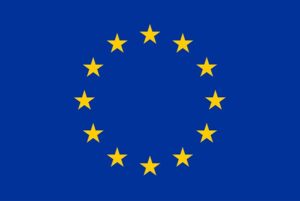
Discover more in this video about the main tasks of MLEAP
The research objectives and expected outcome
The project deals with the approval of machine learning (ML) technology for systems intended for use in safety-related applications in all domains covered by the EASA Basic Regulation (Regulation (EU) 2018/1139).
Data-driven learning techniques are a major opportunity for the aviation industry but come also with a significant number of challenges with respect to the trustworthiness of ML and deep learning (DL) solutions.
EASA published its Artificial Intelligence Roadmap in February 2020, followed by a first major deliverable, a Concept Paper ‘First usable guidance for level 1 machine learning applications’ in April 2021. This concept paper lays down the basis of EASA future guidance for ML applications approval and identifies a number of areas in which further research is necessary to identify efficient and practicable means of compliance with the defined ‘AI trustworthiness’ objectives.
The intended short-term effect of this project will be to streamline the certification and approval processes by identifying concrete means of compliance with the learning assurance objectives of the EASA guidance for ML applications (levels 1, 2 and 3 as defined in the EASA AI Roadmap), with a specific focus on Level 1B and Level 2.
The achieved medium-term effect of the project will be to alleviate some remaining limitations on the acceptance of ML applications in safety-critical applications.
The requested output
The research results will be a set of reports identifying a set of methods and tools to address the following three important topics:
- Guarantees on ‘machine learning model generalisation’
- Guarantees on ‘Data completeness and representativeness’
- Guarantees on algorithm and model robustness
Along with the project, at least one real-scale aviation use case will be used and tested to demonstrate the effectivity and usability of the proposed methods and tools. To demonstrate the relevance, the reliability and the robustness of these tools, some software and hardware environment assessments will be processed.
Airbus Protect – Stakeholders day conference MLEAP Project #3
Airbus Protect hosted the 3rd edition of the Stakeholders Day Conference regarding the R&T project called MLEAP (Machine Learning Application Approval) dealing with Artificial Intelligence. Airbus Protect and its partners presented the on-going development and the latest outcomes of the project to its Stakeholders. The MLEAP team demonstrated the latest achievements and perspectives on the 3 tasks, presenting the selected use cases, the applied methods, and the results analysis of a variety of algorithm and tools for AI use in aeronautics. They focused on investigations of the main elements of an AI workflow: data completeness & representativeness, models generalization assessment, and their robustness and performance stability. Finally, the team showed how the different methods apply to each case, analyzing their behavior, giving some preliminary conclusions about data properties and model performance, and outlining the main perspectives for the remaining work.
This day allowed the stakeholders to meet and exchange with the partners of the project and answer on-going questions about this R&T project.
The recording of the conference and the slides presented during the event are now available :
The slides of the presentation: https://www.protect.airbus.com/wp-content/uploads/2023/05/AP-Stakeholders-day-conference-3-PDF.pdf
The recording video on YouTube: https://www.youtube.com/watch?v=YWaVQL0D5CM
For more information about the project
Information on the progress of this research project will be published on EASA’s MLEAP project page.
- Share